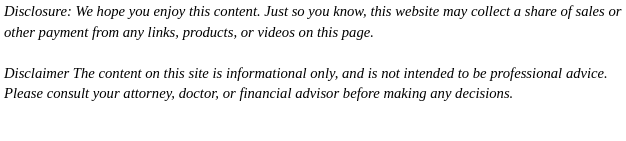
As the amount of linked digital information grows exponentially, older forms of relational databases are unable to handle the sheer volume and interconnectedness of big data sets. New data analytics techniques like text analytics and semantic entity extraction can be used by organizations and agencies to improve their decision making and strategies. Decision makers and such as solution architects, software and systems engineers, and project managers can all use text and semantic entity analytics in fields as diverse as financial compliance and big data analysis cyber security.
Big data is here
Big data is here, and it’s growing rapidly. By 2020, the size of the digital universe will have reached 40 ZB, according to IDC. At the present time, it is estimated that about 1.7 MB of new digital information is being generated every second of every day for each person. This includes social media, marketing information, and more, all derived from online transactions.
This data can be used by businesses and governments agencies to improve their decision making and strategic planning. However, only about 1% of all digital data is ever analyzed. That’s because traditional relational databases can’t handle the volume and complexity of big data. New forms of data analysis, such as text analytics and semantic entity extraction, can be used by businesses to improve productivity, compliance as well as overall strategy.
Data analytics for big data
Big data analysis includes qualitative and quantitative techniques, such as text mining and semantic entity extraction. Digital data is used to analyze behavioral data and patterns. This could include user preferences, community interests and market segments such as demographics, age or gender. Often derived through social media monitoring, it can help businesses to understand consumer sentiment regarding the company, its products and the competition.
These technique can analyze both structured and unstructured text as well as information contained in multiple data sets in different formats. It can also handle the high volumes of data available online.
- Text mining
Text mining is a qualitative process of analyzing large bodies of digital data, including unstructured text such as customer opinions, product reviews, feedback, and sentiment analysis. Text mining consists of four stages: information retrieval, natural language processing, information extraction and data mining. It can help businesses to gain accurate insights into risk, compliance, and threat detection. Natural language processing generates insights into customers preferences and behavior. - Entity extraction
Semantic entity extraction analyzes multiple data sets to find, link, and resolve mentions of the same entity. It performs the identity resolution tasks of deduplication, record linkage, and canonicalization. This can be used by financial institutions for compliance and screening of both customers and employees.
The amount of digital data is increasing rapidly and new data analysis tools are needed to extract useful insights from these. Technique such as text mining and semantic entity resolution can be used to analyze structured and unstructured data that can be used by business and agencies for better decision making and strategic planning.